Correct Disc Golf Form: Classification of the Backhand Throw using Neural Networks
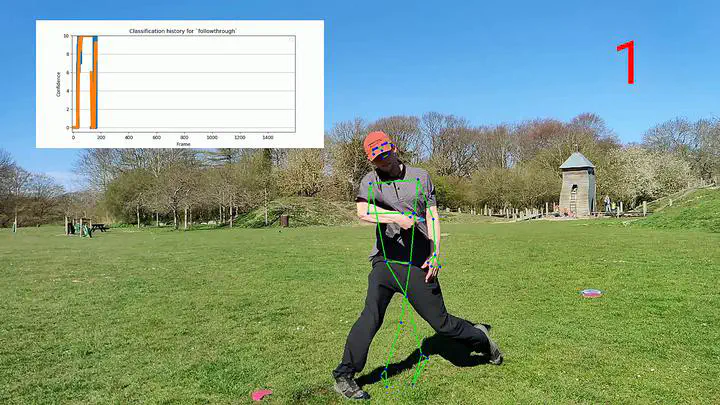
Abstract
Form is essential when analyzing and reviewing a backhand disc golf throw. The form defines if the throw is performed correctly and the poses of the body define the form. By looking at the body poses the throw can be classified, critiqued, and improved upon. The form consists of different motions which are analyzed using 3D data collected using machine learning solutions on a data set of recorded disc golf throws. By processing the 3D data from recorded throws the form is classified into three classes that represent the start, mid, and end of the throw. The three classes are shown as clusters using Principal Component Analysis (PCA). The PCA showed more overlapping clusters for the start and middle of the throw compared to the end. Classification solutions include a variation of trained LSTM networks and a solution using MediaPipe Pose Classification. The paper concludes that LSTM models perform faster and more accurately than the solution using MediaPipe Pose Classification when analyzing disc golf throws. However, the classification only provides insight for classifying the different forms and not the quality of form.